Freeing the Black Swan— AI comes to financial risk management
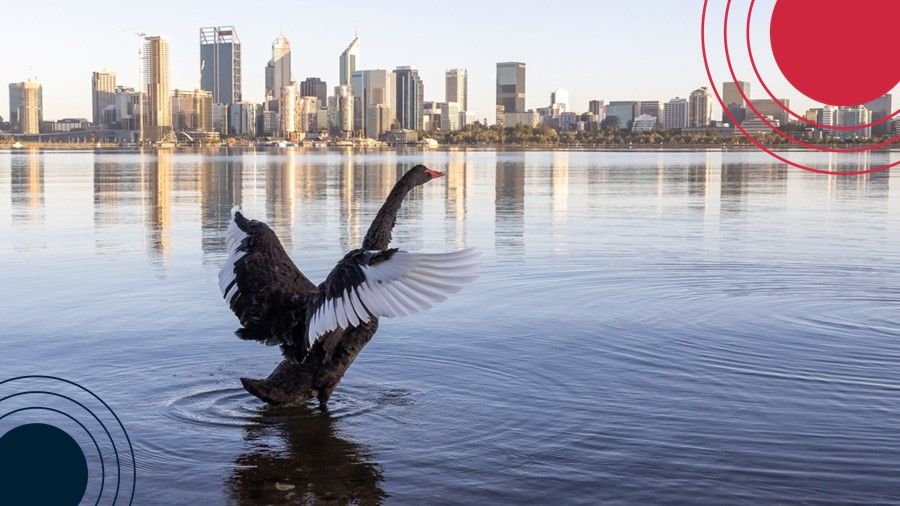
Uncertainty comes with living. It is the unknown and incomputable future. It harkens back to Heisenberg’s uncertainty theorem in physics. The theorem has a long and venerable history, which began with the famous quip between Heisenberg and Einstein. In response to the idea that there were limits on our knowledge as described by the uncertainty theorem, Einstein was famously quoted as saying that “God does not play dice with the universe.” At this point in time, however, Einstein clearly appears to be wrong and there is a fundamental uncertainty in the world.
The uncertainty theorem generally applies to things that are very small, which is why we don’t focus on them. Instead, we deal with risk. That too comes with living, but risk can be calculated. That is, risks can be priced. That is why we worry about risk management and not uncertainty management.
Risk is model dependent
The field of risk management needs risk models which employ probability distributions. While there is a plethora of such models and distributions in practice, they virtually all emanate from the work of the late Harry Markowitz. Markowitz was a winner of the Jon Von Neumann Theory Prize which could not speak higher of Markowitz’s contribution to finance.
Markowitz’s fundamental model came from the simple notion that a person doesn’t put all their eggs in one basket. He envisioned risk in a world of portfolios defined by the mean and variance of their returns.
The application of Markowitz’s model required empirical testing. Extensive data fitting stood between the mean variance idea, and the risk management we know of with its stalwarts of Value at Risk (VaR) and its conditional cousin CVaR. The test of Markowitz’s model required computers and data.
A critical, and underappreciated role here was the computerization of stock market prices. Models without data lack validation and Harry Markowitz’s idea needed data, lots of data to prove it.
Markowitz published his seminal work, "Portfolio Selection" in The Journal of Finance in March 1952. Then there were few options for a thorough empirical testing of the theory. That came much later with the creation of the CRSP data tapes at the University of Chicago.
Data makes the model
In March 1960 the Center for Research in Security Prices was created. Merrill Lynch funded the collection of stock data from the major exchanges as far back as the 1929 Crash. That data set is now widely used in academics and was instrumental in computerized testing of what is now the Capital Asset Pricing Model (CAPM).
Both the data and the model have many limitations, nevertheless, they have combined to advance our understanding of securities pricing.
For example, when CAPM was verified in 1972 by Black et al. in the paper titled “The Capital Asset Pricing Model: Some Empirical Tests” it was back-tested with New York Stock Exchange (NYSE) monthly data. Two decades later, when Fama and French published the famous three-factor models, they used a large dataset that covered the NYSE, AMEX, and NASDAQ stock universe and spanned 27 years. In 2015, when they came up with the five-factor model to better explain the risk premiums, they used a more comprehensive dataset with 50 years of history ending in 2013.
However, the 2013 data was merely 1/10th of the 2022 volume. In addition, while the price series on equities has been enriched with other data sources, the factor risk model is fundamentally only about prices. And we are often unsatisfied with the results of the model. The financial markets have become more complicated which compels us to use more sensitive and comprehensive real-time data to build more relevant risk models.
Stationary distribution assumption
One core assumption of the factor risk and VaR models is that the data series being modeled are stationary. That is, the properties of data series do not change over time.
However, in our recent period of high interest rates and deepening yield curve inversions, stable markets may continue to be elusive, as the recent large bank failures serve to emphasize.
And financial risk, in absolute terms, are growing as economies grow and novel risks emerge. The U.S. GDP in 1952, when Markowitz wrote, was about $0.4 T and now it is $26.5 T. These are nominal data but serve to illustrate that if risk metrics were important in 1952, they are more so now.
The novelty of the emerging risks is as important as the growth in the amount of assets at risk. The range of seemingly independent macroeconomic risks is now staggering. The shortlist is the return of a pandemic, global warming, the collapse of a major country, and many more. The point is that we can expect the core assumptions of factor risk and VaR models to be violated even more frequently.
AI risk models come to the fore
AI models are potential game changers in the morass of risk. First, AI models allow each application of the AI to generate its own unique solution under different market conditions for a particular security. Understanding the model is complex, of course, and we will talk about the explainable AI area, which disentangles AI models in a later article.
AI modeling offers a powerful new approach to risk management. But the results of an AI risk analysis cannot be so foreign that it is inexplicable to analysts. AI can produce versions of current risk parameters, which are easily digestible. One such measure we have generated provides unique risk measures of this sort with AI generated forward-looking VaRs. This exploits AI’s unique ability to pattern match current events with only relevant, not all, historical observations, which relaxes the data stationarity assumption.
And finally, the critical role of data and data consolidation becomes clear. For an AI model, the more it knows, the better it can predict. But vast troves of data soon become unmanageable. The AI is far more capable than the factor risk model at capturing the multi-dimensional nature of current financial risks. But this can only be practical with efficient indicator aggregation.
Data aggregation is one of our specialties and makes AI models efficient in risk management. IndicatorLab’s solution can make the data aggregation process easier than before through a patent-pending additive design.
AI redefines risk management
The emergence of AI models is redefining risk management. Risk becomes individually managed with uniquely fit models for each security. The fit model then becomes forward-looking with the right algorithms. It can also absorb the new data from emerging risks to fill in the tails of any distribution. Well-fit AI risk models fill in the tails of our current model estimation. AI offers the chance to build models that can free the black swans trapped in tails of our limited VaR models.
About the Authors
Dr. Philip Fischer and eBooleant Consulting LLC
eBooleant Consulting LLC is an economic and financial consulting company focusing on fintech, AI, risk management, and public policy founded by Dr. Philip Fischer. The company also offers expert witness, training, and teaching as well as serving in special advisory roles. Learn more at ebooleant.com.
Dr. Rein Wu and IndicatorLab
IndicatorLab is an AI-driven, no-code financial information aggregator specializing in investment strategy creation and risk analysis founded by Dr. Rein Wu, Dr. Jason, and Dr. Yun. IndicatorLab offers a unique combination of customization, transparency, and downside protection through an AI SaaS platform that can solve risk problems in real time. Its platform ability to provide forward looking VaR will reinvent risk management for portfolio managers, traders, and financial analysts. For more information, visit indicatorlab.xyz.
Spread the word
Tweet this: Free the Black Swan - AI comes to financial risk management, a new article by Dr. Philip J. Fischer of @ebooleant and Dr. Rein Wu of @IndicatorLab Fintech #blackswan #AI #Trading #RiskManagement #DataAnalytics #WallStreet #InvestmentStrategy #Portfoliomanagement