Corporate AI doesn’t need to compete with ChatGPT
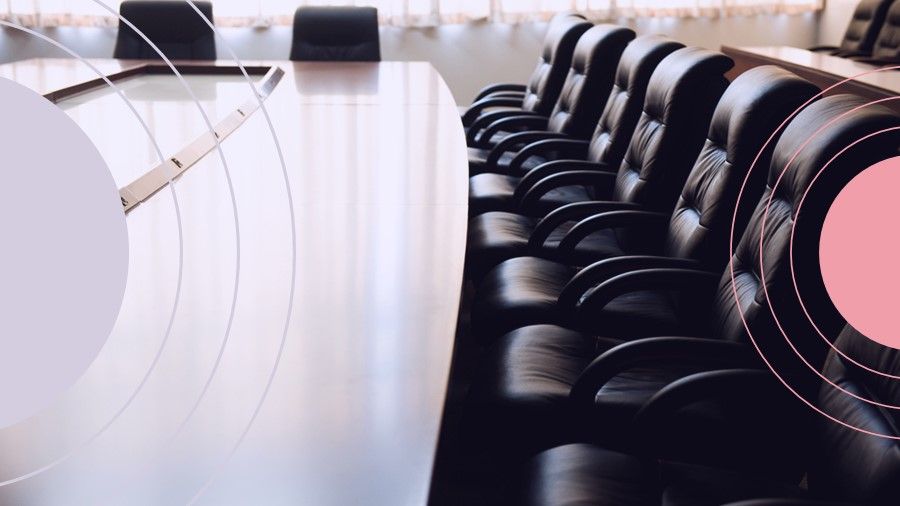
The business of secrets is technologically intense. An afternoon at The SPYSCAPE museum in New York City humbles a person with its secrets and the machines invented to figure them out—machines like the WWII German Enigma machines which turned ultra secret Nazi orders into code. The Enigma machines creating the code were small typewriters that required a room-sized vacuum tube computer to help decode their messages.
The computers, however, kept getting bigger, until they earned the name of “Colossus.”[1]The whole experience reminded me of the path we are taking in AI and ML where the sheer size of our solutions becomes an issue itself.
The decoding process developed by the British code breakers, including the likes of Alan Turing, is analogous to the one we are on in AI. The process of creating machine intelligence today involves taking data inputs as enigmatic as the Enigma machine ever made and making sense of them. Data with secrets are supplied to computer algorithms seeking to discern an underlying meaning. The feverish competition of AI groups is not quite at war-level, although the international competition may have some of that intensity. In AI, just as in WWII, time is of the essence.
The drive to more intelligent models
The rise in computing prowess in AI has grown at a spectacular rate. Moore’s Law estimates that computer capacity would double every two years. That now seems passe. The doubling time for large AI models has been on the order of months not years.
One would think that this rise in machine efficiency would lead to smaller, more efficient models. After all, improvements in hardware have occurred causing the cost per operation to fall.[2]But, ever more chips are added, and customers are willing to pay for it.
This phenomenal rate of growth in the size of AI solutions seems clearly unsustainable in the long run.[3]But the size of AI models has to date been maintained by the big models. The largest models have grown faster in size than intermediate sized models.[4]
The demand for larger models comes from their obvious advantages. Large AI and ML models have demonstrated improved accuracy and better performance with every evolution. The international race to generalized machine intelligence is an important force driving greater model size.
But with size comes limitations. Larger models are produced at greater cost. In addition, running them creates accelerating energy consumption and is associated with increased carbon footprints. Large models can offer unique ethical considerations involving bias and fairness, as well as privacy issues.
The juggernaut to larger models looks to continue anyway but at a cost. The result is that users must adapt to exploit model strengths while controlling costs. The process is not without its critics.
OpenAI’s CEO Sam Altman maintains that larger models have already run their course and that “giant, giant models,” are not the future. Instead, novel approaches to AI will evolve.[5]
We will have to wait to see if there is a halt or even a pause towards ever more giant models. Meanwhile firms are left with businesses to run, and the already huge size of the leading models may be beyond their control. Some of the AI models that are most important to the firm, however, are in their control to develop and integrate into their data and analytic systems.
Corporate strategies for dealing with model size
The first answer about corporate applications of AI is that companies live in a veritable sea of emerging technologies, many of which are AI. Large corporate vendors like Salesforce and Bloomberg, to say nothing of Amazon, Google, and Microsoft, are sprinting to put AI applications into virtually every one of their applications. So, it is not a question of whether businesses will have to implement AI, in many cases it’s being done for them.
Many businesses nevertheless will have to do the bulk of the most valuable AI work themselves since they have proprietary processes and data. Here model choice and size are in their control. Corporations do not have to create new models to compete with ChatGPT. They have unique business processes, amenable to automated decision making which can use smaller specialized models.
And businesses are getting some help in choosing where AI and ML will work best. A lot of advanced planning has now been done to identify areas where AI will generally be most useful. We can now use the results of public studies for planning purposes. A company can skip some of the experimentation that might have been necessary even a year ago.
There are now a long list of use cases showing AI’s ability to enhance productivity in areas like programming, writing, human relations and marketing that AI applications. These areas should be an immediate consideration. And specialized models can be efficiently employed.[6]
A company needs to think through each AI application carefully to ensure that the best model and system design are employed, but it doesn't need to speculate about the potential benefits. And for most, the size of the model employed, though often large, will not be beyond a firm’s current system capabilities. And larger models are not necessarily smarter.[7]
Fine-tuning
A gargantuan model isn't necessarily better at addressing the specialized tasks or unique datasets that a new organization might have. Fine-tuning a smaller, pre-trained model to adapt to specific organizational needs can yield highly effective results, often with a significantly smaller carbon footprint and at a fraction of the cost.
The trade-off between model size and performance isn't linear; past a certain point, the gains in performance are marginal compared to the increase in resource allocation. Therefore, smaller models that are fine-tuned for specialized tasks offer a more sustainable, efficient, and economically viable pathway for new organizations to harness the power of AI. This approach enables them to be competitive without entering an arms race of computational power and model size, allowing them to focus on innovation and rapid deployment to meet business goals.
In addition, the size of the AI and ML models for specialized tasks is controllable. Cleaning up and preprocessing data inputs can greatly reduce model size and training time.
Unique data, unique models
Systems architecture is also critical to managing models. Cost can dictate against Internet centralized cloud-based designs without efficient systems design. But SaaS style solutions allow other advantages such as lower personnel costs.
As organizations grapple with managing the sheer size and computational demands of AI/ML models, the advent of vector databases presents a promising avenue for operational efficiency. These specialized databases allow companies to vectorize their proprietary data, turning complex information into a mathematical format that can be quickly and precisely queried.
Unlike traditional databases that may struggle with high-dimensional data and intricate queries, vector databases excel in handling such complexities, offering a more efficient use of computational resources. The ability to vectorize proprietary data allows companies to better match specific queries to their unique datasets, thereby eliminating the need to rely on excessively large models to handle specialized tasks.
Essentially, it's a smarter way of utilizing the data, allowing the AI model to focus solely on the task at hand rather than navigating through vast, irrelevant data points. This approach not only brings down computational costs but also speeds up the time-to-insight, a critical factor in today’s fast-paced business landscape. In this framework, the challenge of "size" shifts from the AI models to the underlying databases, which are specifically designed to manage high-dimensional data efficiently. Companies that invest in vector databases find themselves better positioned to tackle the challenges of today’s data-intensive environments, all while maintaining a lean, more sustainable operational model. This could be the practical "transistor moment" for many firms, allowing them to maximize AI/ML benefits without spiraling costs and complexities, much like the transition from the room-sized Colossus to today's more manageable computing systems.
Size is today’s problem
Bigger models are often a preference. But they can be costly to build, train and run. For many companies, it is not a matter of automating or not. It is much more a matter of finding an appropriate firm-compatible, cost-efficient choice. Here, minimizing, not maximizing size, is the challenge. As many analysts now say, we will be forced to large, but not necessarily very large models.
The spy museum trek does offer another hope. The Colossus vacuum tube computer is now in a museum. Shrinking the Colossus to today’s dimensions for a practical computer required the invention of the transistor. We may have to wait for the evolution of the quantum computer. In between, firms need to constrain the natural growth tendencies of AI/ML models where possible. Leaner models which lower energy costs may be better.
About the Authors
Dr. Philip Fischer and eBooleant Consulting LLC
eBooleant Consulting LLC is an economic and financial consulting company focusing on fintech, AI, risk management, and public policy founded by Dr. Philip Fischer. The company also offers expert witness, training, and teaching as well as serving in special advisory roles to startups. Learn more at ebooleant.com.
Dr. Rein Wu and IndicatorLab
IndicatorLab is an AI-driven, no-code financial information aggregator specializing in investment strategy creation and risk analysis founded by Dr. Rein Wu, Dr. Jason, and Dr. Yun. IndicatorLab offers a unique combination of customization, transparency, and downside protection through an AI SaaS platform that can solve risk problems in real time. Its platform ability to provide forward looking VaR will reinvent risk management for portfolio managers, traders, and financial analysts. For more information, visit indicatorlab.xyz.
[1]https://en.wikipedia.org/wiki/Colossus_computer
[2]https://arxiv.org/abs/2202.05924
[3]https://openai.com/research/ai-and-compute
[4]https://arxiv.org/pdf/2207.02852.pdf
[5]https://www.wired.com/story/openai-ceo-sam-altman-the-age-of-giant-ai-models-is-already-over/#:~:text=The%20stunning%20capabilities%20of%20ChatGPT%2C%20the%20chatbot%20from,unclear%20exactly%20where%20future%20advances%20will%20come%20from.
[6]https://www.nytimes.com/2023/03/28/business/economy/jobs-ai-artificial-intelligence-chatgpt.html?unlocked_article_code=vQxcJrIOtpQEqAQJVZTdCe2wpEgm1U2bwCQndU_62mLjmlbTfSR2NdYeuHDtQhdvPBlzOiyqS5WQgCYCnGh_-0GeSTIhhg3aOVpfoNRGoTysO1WehfplPLMKH5tLLWMJYQPNGxN9P6-oljGXd8Tb8vCjUfUdT7kF2Sk2AJn3ihs7MSlGbCEoSFGGEhczxzrdUjywi64Nzu0zZFGi4VB4np7gMDSl9r9ZZx_JJxgKRrSNJxp759k60so1bjGlBy4WrUgpwsUdxPi2tHPXXdoVk5OxqU7_FHVT5DrQsmO2iKX2mYxfoo2vLNOjDtS3wFWMjvLJW9ocHf5078gLOsme9zCbULn-4b7Rec9zQ7ToQXPkN_ewAXYXKOqK&smid=url-share